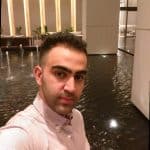
Every day, consumers are exposed to tons of digital contents on different platforms and screens and this has increased their expectation to receive more personalised and related contents because we all could agree that being exposed to non-relevant contents can be really annoying. Marketers, on the other hand, do not want to show contents to irrelevant consumer segments as well because it is simply a waste of resources.
Well, Thanks to machine learning (ML), marketers can now show tailor-made ads to relevant consumer segments to maximise results. By the end of this article, you will have a good understanding of what ML is and how it can be used for personalisation. Bear in mind that the road to personalisation can be long and challenging but once it is done right, it can significantly optimise your digital marketing efforts.
In simple terms, ML is a way to quickly label and analyse a large data set. ML can help us find undetectable patterns and predict future outcomes. Although humans can do this on their own, a machine, on the other hand, can do it much faster and on an extremely larger scale. You can either build your own machine learning program from scratch which requires lots of upfront investment and expertise or you can leverage on marketing products from companies such as Google which already have ML integrated into them.
The first step to make ML works for your organisation is to collect the data. Your data might exist across different platforms such as sales, customer service and CRM. Once you have all your data in one place, you need to clean and organise it. Remember, the quality of your ML results depends on the quality of your data. Gathering your data will enable you to better understand and respond to your customer needs which ultimately leads to growth.
The second step is to analyse the data which you have already integrated. In this step, you want to build an accurate model which you can rely on. For example, you can build a model to predict the probability of purchasing a specific product by providing millions of examples of previous customers who have bought that product as well as those who have not. The more high-quality data you feed to your model, the more accurate the prediction will be.
Once you have a reliable model, the third step is activation where you can take the output and use it to segment your customer. Once customer segmentation is done, you have a clear view of your customer base and thus, you can provide personalised offers to each customer segment which helps you wisely plan where and how to spend your budget. This will also help you to reach out to those consumers that you want to reach and avoid wasting resources on those who are not interested in what you are offering.
In the next article, I will talk more about how to prepare your organisation for ML journey to take maximum advantage of this great technology and also introduce some Google ML Products which you can explore and utilize them for your business.
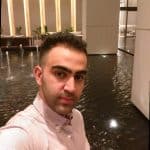
Amir is currently working as a Google Analytics engineer at Kasatria Technologies in Kuala Lumpur. Prior to this, he was an analytics consultant at Artefact Malaysia. He holds a Master of Business Administration in International Marketing from Coventry University (UK) and has great interest in digital marketing, particularly digital analytics and big data. Follow him on LinkedIn.
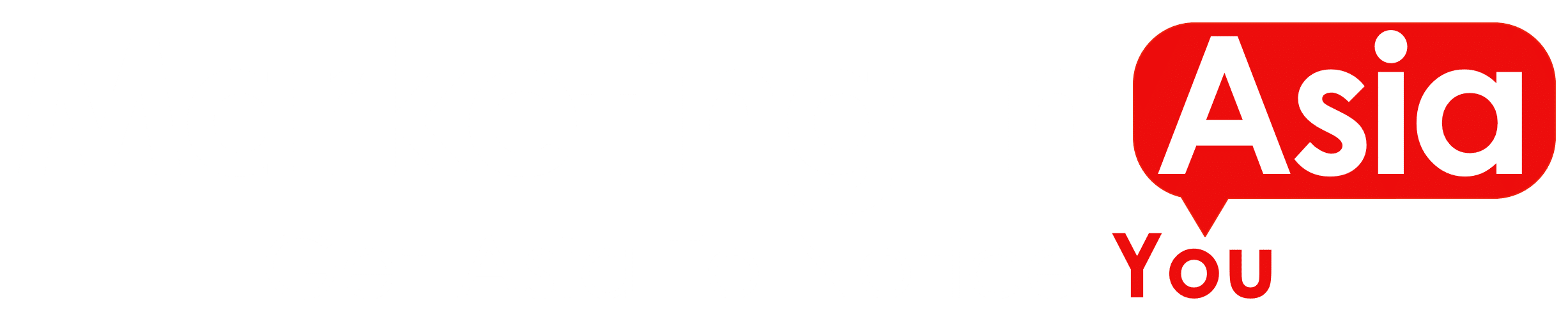